Exploratory Regression
أداة الانحدار
الاستكشافي
ArcMap
ArcGIS
How to use Exploratory
Regression Tool in Arc Toolbox??
 |
Exploratory Regression Tool |
كيفية استخدام أداة الانحدار الاستكشافي ؟؟
Path to access the toolمسار الوصول الى الأداة
:
Exploratory Regression Tool, Modeling
Spatial Relationships Toolset, Spatial Statistics Tools Toolbox
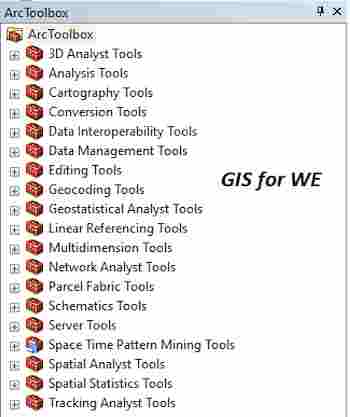
Exploratory Regression
The Exploratory
Regression tool evaluates all possible combinations of the input candidate
explanatory variables, looking for OLS models that best explain the dependent
variable within the context of user-specified criteria.
You can access the
results of this tool (including the optional report file) from the Results
window. If you disable background processing, results will also be written to
the Progress dialog box.
تقوم أداة Exploratory Regression
بتقييم جميع المجموعات الممكنة من المتغيرات التوضيحية لمرشح الإدخال ، وتبحث عن
نماذج OLS التي تشرح بشكل أفضل المتغير التابع في سياق المعايير المحددة من
قبل المستخدم.
يمكنك الوصول إلى نتائج هذه الأداة (بما في ذلك
ملف التقرير الاختياري) من نافذة النتائج. إذا قمت بتعطيل معالجة الخلفية ، فستتم
أيضًا كتابة النتائج في مربع حوار التقدم.
1. Input Features ادخل المعالم
The feature class or
feature layer containing the dependent and candidate explanatory variables to
analyze.
فئة المعالم أو طبقة المعالم التي تحتوي على
المتغيرات التوضيحية التابعة والمرشحة لتحليلها.
Dependent Variable
The numeric field containing the observed values you want to model using
OLS.
Candidate Explanatory Variables
A list of fields to try as OLS model explanatory variables.
Weights Matrix File (optional)
A file containing spatial weights that define the spatial relationships
among your input features. This file is used to assess spatial autocorrelation
among regression residuals. You can use the Generate Spatial Weights Matrix
File tool to create this. When you do not provide a spatial weights matrix
file, residuals are assessed for spatial autocorrelation based on each
feature's 8 nearest neighbors.
Note: The spatial weights matrix file is only used to analyze spatial
structure in model residuals; it is not used to build or to calibrate any of
the OLS models.
Output Report File (optional)
The report file contains tool results, including details about any models
found that passed all the search criteria you entered. This output file also
contains diagnostics to help you fix common regression problems in the case
that you don't find any passing models.
Output Results Table (optional)
The optional output table created containing the explanatory variables and
diagnostics for all of the models within the Coefficient p-value and VIF value
cutoffs.
Maximum Number of Explanatory
Variables (optional)
All models with explanatory variables up to the value entered here will be
assessed. If, for example, the Minimum Number of Explanatory Variables is 2 and
the Maximum Number of Explanatory Variables is 3, the Exploratory Regression
tool will try all models with every combination of two explanatory variables,
and all models with every combination of three explanatory variables.
Minimum Number of Explanatory
Variables (optional)
This value represents the minimum number of explanatory variables for
models evaluated. If, for example, the Minimum Number of Explanatory Variables
is 2 and the Maximum Number of Explanatory Variables is 3, the Exploratory
Regression tool will try all models with every combination of two explanatory
variables, and all models with every combination of three explanatory
variables.
Minimum Acceptable Adj R Squared
(optional)
This is the lowest Adjusted R-Squared value you consider a passing model.
If a model passes all of your other search criteria, but has an Adjusted
R-Squared value smaller than the value entered here, it will not show up as a
Passing Model in the Output Report File. Valid values for this parameter range
from 0.0 to 1.0. The default value is 0.05, indicating that passing models will
explain at least 50 percent of the variation in the dependent variable.
Maximum Coefficient p value Cutoff
(optional)
For each model evaluated, OLS computes explanatory variable coefficient
p-values. The cutoff p-value you enter here represents the confidence level you
require for all coefficients in the model in order to consider the model passing.
Small p-values reflect a stronger confidence level. Valid values for this
parameter range from 1.0 down to 0.0, but will most likely be 0.1, 0.05, 0.01,
0.001, and so on. The default value is 0.05, indicating passing models will
only contain explanatory variables whose coefficients are statistically at the
95 percent confidence level (p-values smaller than 0.05). To relax this default
you would enter a larger p-value cutoff, such as 0.1. If you are getting lots
of passing models, you will likely want to make this search criteria more
stringent by decreasing the default p-value cutoff from 0.05 to 0.01 or
smaller.
Maximum VIF Value Cutoff
(optional)
This value reflects how much redundancy (multicollinearity) among model
explanatory variables you will tolerate. When the VIF (Variance Inflation
Factor) value is higher than about 7.5, multicollinearity can make a model
unstable; consequently, 7.5 is the default value here. If you want your passing
models to have less redundancy, you would enter a smaller value, such as 5.0,
for this parameter.
Minimum Acceptable Jarque Bera p
value (optional)
The p-value returned by the Jarque-Bera diagnostic test indicates whether
the model residuals are normally distributed. If the p-value is statistically
significant (small), the model residuals are not normal and the model is
biased. Passing models should have large Jarque-Bera p-values. The default
minimum acceptable p-value is 0.1. Only models returning p-values larger than
this minimum will be considered passing. If you are having trouble finding
unbiased passing models, and decide to relax this criterion, you might enter a
smaller minimum p-value such as 0.05.
Minimum Acceptable Spatial
Autocorrelation p value (optional)
For models that pass all of the other search criteria, the Exploratory
Regression tool will check model residuals for spatial clustering using Global
Moran's I. When the p-value for this diagnostic test is statistically
significant (small), it indicates the model is very likely missing key explanatory
variables (it isn't telling the whole story). Unfortunately, if you have
spatial autocorrelation in your regression residuals, your model is
misspecified, so you cannot trust your results. Passing models should have
large p-values for this diagnostic test. The default minimum p-value is 0.1.
Only models returning p-values larger than this minimum will be considered
passing. If you are having trouble finding properly specified models because of
this diagnostic test, and decide to relax this search criteria, you might enter
a smaller minimum such as 0.05.
2.
Dependent Variable المتغير التابع
The numeric field
containing the observed values you want to model using OLS.
الحقل الرقمي الذي يحتوي على القيم المرصودة التي
تريد تصميمها باستخدام OLS.
Candidate Explanatory Variables
A list of fields to try as OLS model explanatory variables.
Weights Matrix File (optional)
A file containing spatial weights that define the spatial relationships
among your input features. This file is used to assess spatial autocorrelation
among regression residuals. You can use the Generate Spatial Weights Matrix
File tool to create this. When you do not provide a spatial weights matrix
file, residuals are assessed for spatial autocorrelation based on each
feature's 8 nearest neighbors.
Note: The spatial weights matrix file is only used to analyze spatial
structure in model residuals; it is not used to build or to calibrate any of
the OLS models.
Output Report File (optional)
The report file contains tool results, including details about any models
found that passed all the search criteria you entered. This output file also
contains diagnostics to help you fix common regression problems in the case
that you don't find any passing models.
Output Results Table (optional)
The optional output table created containing the explanatory variables and
diagnostics for all of the models within the Coefficient p-value and VIF value
cutoffs.
Maximum Number of Explanatory
Variables (optional)
All models with explanatory variables up to the value entered here will be
assessed. If, for example, the Minimum Number of Explanatory Variables is 2 and
the Maximum Number of Explanatory Variables is 3, the Exploratory Regression
tool will try all models with every combination of two explanatory variables,
and all models with every combination of three explanatory variables.
Minimum Number of Explanatory
Variables (optional)
This value represents the minimum number of explanatory variables for
models evaluated. If, for example, the Minimum Number of Explanatory Variables
is 2 and the Maximum Number of Explanatory Variables is 3, the Exploratory
Regression tool will try all models with every combination of two explanatory
variables, and all models with every combination of three explanatory
variables.
Minimum Acceptable Adj R Squared
(optional)
This is the lowest Adjusted R-Squared value you consider a passing model.
If a model passes all of your other search criteria, but has an Adjusted
R-Squared value smaller than the value entered here, it will not show up as a
Passing Model in the Output Report File. Valid values for this parameter range
from 0.0 to 1.0. The default value is 0.05, indicating that passing models will
explain at least 50 percent of the variation in the dependent variable.
Maximum Coefficient p value Cutoff
(optional)
For each model evaluated, OLS computes explanatory variable coefficient
p-values. The cutoff p-value you enter here represents the confidence level you
require for all coefficients in the model in order to consider the model
passing. Small p-values reflect a stronger confidence level. Valid values for
this parameter range from 1.0 down to 0.0, but will most likely be 0.1, 0.05,
0.01, 0.001, and so on. The default value is 0.05, indicating passing models
will only contain explanatory variables whose coefficients are statistically at
the 95 percent confidence level (p-values smaller than 0.05). To relax this
default you would enter a larger p-value cutoff, such as 0.1. If you are
getting lots of passing models, you will likely want to make this search
criteria more stringent by decreasing the default p-value cutoff from 0.05 to
0.01 or smaller.
Maximum VIF Value Cutoff
(optional)
This value reflects how much redundancy (multicollinearity) among model
explanatory variables you will tolerate. When the VIF (Variance Inflation
Factor) value is higher than about 7.5, multicollinearity can make a model
unstable; consequently, 7.5 is the default value here. If you want your passing
models to have less redundancy, you would enter a smaller value, such as 5.0,
for this parameter.
Minimum Acceptable Jarque Bera p
value (optional)
The p-value returned by the Jarque-Bera diagnostic test indicates whether
the model residuals are normally distributed. If the p-value is statistically
significant (small), the model residuals are not normal and the model is
biased. Passing models should have large Jarque-Bera p-values. The default
minimum acceptable p-value is 0.1. Only models returning p-values larger than
this minimum will be considered passing. If you are having trouble finding
unbiased passing models, and decide to relax this criterion, you might enter a
smaller minimum p-value such as 0.05.
Minimum Acceptable Spatial
Autocorrelation p value (optional)
For models that pass all of the other search criteria, the Exploratory
Regression tool will check model residuals for spatial clustering using Global
Moran's I. When the p-value for this diagnostic test is statistically
significant (small), it indicates the model is very likely missing key
explanatory variables (it isn't telling the whole story). Unfortunately, if you
have spatial autocorrelation in your regression residuals, your model is
misspecified, so you cannot trust your results. Passing models should have
large p-values for this diagnostic test. The default minimum p-value is 0.1.
Only models returning p-values larger than this minimum will be considered
passing. If you are having trouble finding properly specified models because of
this diagnostic test, and decide to relax this search criteria, you might enter
a smaller minimum such as 0.05.
3.
Candidate Explanatory Variables المتغيرات
التوضيحية للمرشح
A list of fields to try
as OLS model explanatory variables.
قائمة الحقول التي يجب تجربتها كمتغيرات توضيحية
لنموذج OLS.
Weights Matrix File (optional)
A file containing spatial weights that define the spatial relationships
among your input features. This file is used to assess spatial autocorrelation
among regression residuals. You can use the Generate Spatial Weights Matrix
File tool to create this. When you do not provide a spatial weights matrix
file, residuals are assessed for spatial autocorrelation based on each
feature's 8 nearest neighbors.
Note: The spatial weights matrix file is only used to analyze spatial
structure in model residuals; it is not used to build or to calibrate any of
the OLS models.
Output Report File (optional)
The report file contains tool results, including details about any models
found that passed all the search criteria you entered. This output file also
contains diagnostics to help you fix common regression problems in the case
that you don't find any passing models.
Output Results Table (optional)
The optional output table created containing the explanatory variables and
diagnostics for all of the models within the Coefficient p-value and VIF value
cutoffs.
Maximum Number of Explanatory
Variables (optional)
All models with explanatory variables up to the value entered here will be
assessed. If, for example, the Minimum Number of Explanatory Variables is 2 and
the Maximum Number of Explanatory Variables is 3, the Exploratory Regression
tool will try all models with every combination of two explanatory variables,
and all models with every combination of three explanatory variables.
Minimum Number of Explanatory
Variables (optional)
This value represents the minimum number of explanatory variables for
models evaluated. If, for example, the Minimum Number of Explanatory Variables
is 2 and the Maximum Number of Explanatory Variables is 3, the Exploratory
Regression tool will try all models with every combination of two explanatory
variables, and all models with every combination of three explanatory
variables.
Minimum Acceptable Adj R Squared
(optional)
This is the lowest Adjusted R-Squared value you consider a passing model.
If a model passes all of your other search criteria, but has an Adjusted
R-Squared value smaller than the value entered here, it will not show up as a
Passing Model in the Output Report File. Valid values for this parameter range
from 0.0 to 1.0. The default value is 0.05, indicating that passing models will
explain at least 50 percent of the variation in the dependent variable.
Maximum Coefficient p value Cutoff
(optional)
For each model evaluated, OLS computes explanatory variable coefficient
p-values. The cutoff p-value you enter here represents the confidence level you
require for all coefficients in the model in order to consider the model
passing. Small p-values reflect a stronger confidence level. Valid values for
this parameter range from 1.0 down to 0.0, but will most likely be 0.1, 0.05,
0.01, 0.001, and so on. The default value is 0.05, indicating passing models
will only contain explanatory variables whose coefficients are statistically at
the 95 percent confidence level (p-values smaller than 0.05). To relax this
default you would enter a larger p-value cutoff, such as 0.1. If you are
getting lots of passing models, you will likely want to make this search
criteria more stringent by decreasing the default p-value cutoff from 0.05 to
0.01 or smaller.
Maximum VIF Value Cutoff
(optional)
This value reflects how much redundancy (multicollinearity) among model
explanatory variables you will tolerate. When the VIF (Variance Inflation
Factor) value is higher than about 7.5, multicollinearity can make a model
unstable; consequently, 7.5 is the default value here. If you want your passing
models to have less redundancy, you would enter a smaller value, such as 5.0,
for this parameter.
Minimum Acceptable Jarque Bera p
value (optional)
The p-value returned by the Jarque-Bera diagnostic test indicates whether
the model residuals are normally distributed. If the p-value is statistically
significant (small), the model residuals are not normal and the model is
biased. Passing models should have large Jarque-Bera p-values. The default
minimum acceptable p-value is 0.1. Only models returning p-values larger than
this minimum will be considered passing. If you are having trouble finding
unbiased passing models, and decide to relax this criterion, you might enter a
smaller minimum p-value such as 0.05.
Minimum Acceptable Spatial
Autocorrelation p value (optional)
For models that pass all of the other search criteria, the Exploratory
Regression tool will check model residuals for spatial clustering using Global
Moran's I. When the p-value for this diagnostic test is statistically
significant (small), it indicates the model is very likely missing key
explanatory variables (it isn't telling the whole story). Unfortunately, if you
have spatial autocorrelation in your regression residuals, your model is
misspecified, so you cannot trust your results. Passing models should have
large p-values for this diagnostic test. The default minimum p-value is 0.1.
Only models returning p-values larger than this minimum will be considered
passing. If you are having trouble finding properly specified models because of
this diagnostic test, and decide to relax this search criteria, you might enter
a smaller minimum such as 0.05.
4.
Weights Matrix File (optional) ملف مصفوفة
الأوزان (اختياري)
A file containing
spatial weights that define the spatial relationships among your input
features. This file is used to assess spatial autocorrelation among regression
residuals. You can use the Generate Spatial Weights Matrix File tool to create
this. When you do not provide a spatial weights matrix file, residuals are
assessed for spatial autocorrelation based on each feature's 8 nearest
neighbors.
Note: The spatial
weights matrix file is only used to analyze spatial structure in model
residuals; it is not used to build or to calibrate any of the OLS models.
ملف يحتوي على أوزان مكانية تحدد العلاقات
المكانية بين معالم الإدخال. يستخدم هذا الملف لتقييم الارتباط المكاني الذاتي بين
بقايا الانحدار. يمكنك استخدام أداة إنشاء ملف مصفوفة أوزان مكانية لإنشاء هذا.
عندما لا تقدم ملف مصفوفة للأوزان المكانية ، يتم تقييم القيم المتبقية للارتباط
التلقائي المكاني بناءً على أقرب 8 جيران لكل ميزة.
ملاحظة: ملف مصفوفة الأوزان المكانية يستخدم فقط
لتحليل البنية المكانية في بقايا النموذج ؛ لا يتم استخدامه لبناء أو معايرة أي من
نماذج OLS.
Output Report File (optional)
The report file contains tool results, including details about any models
found that passed all the search criteria you entered. This output file also
contains diagnostics to help you fix common regression problems in the case
that you don't find any passing models.
Output Results Table (optional)
The optional output table created containing the explanatory variables and
diagnostics for all of the models within the Coefficient p-value and VIF value
cutoffs.
Maximum Number of Explanatory
Variables (optional)
All models with explanatory variables up to the value entered here will be
assessed. If, for example, the Minimum Number of Explanatory Variables is 2 and
the Maximum Number of Explanatory Variables is 3, the Exploratory Regression
tool will try all models with every combination of two explanatory variables,
and all models with every combination of three explanatory variables.
Minimum Number of Explanatory
Variables (optional)
This value represents the minimum number of explanatory variables for
models evaluated. If, for example, the Minimum Number of Explanatory Variables
is 2 and the Maximum Number of Explanatory Variables is 3, the Exploratory
Regression tool will try all models with every combination of two explanatory
variables, and all models with every combination of three explanatory
variables.
Minimum Acceptable Adj R Squared
(optional)
This is the lowest Adjusted R-Squared value you consider a passing model.
If a model passes all of your other search criteria, but has an Adjusted
R-Squared value smaller than the value entered here, it will not show up as a
Passing Model in the Output Report File. Valid values for this parameter range
from 0.0 to 1.0. The default value is 0.05, indicating that passing models will
explain at least 50 percent of the variation in the dependent variable.
Maximum Coefficient p value Cutoff
(optional)
For each model evaluated, OLS computes explanatory variable coefficient
p-values. The cutoff p-value you enter here represents the confidence level you
require for all coefficients in the model in order to consider the model
passing. Small p-values reflect a stronger confidence level. Valid values for
this parameter range from 1.0 down to 0.0, but will most likely be 0.1, 0.05,
0.01, 0.001, and so on. The default value is 0.05, indicating passing models
will only contain explanatory variables whose coefficients are statistically at
the 95 percent confidence level (p-values smaller than 0.05). To relax this
default you would enter a larger p-value cutoff, such as 0.1. If you are
getting lots of passing models, you will likely want to make this search
criteria more stringent by decreasing the default p-value cutoff from 0.05 to
0.01 or smaller.
Maximum VIF Value Cutoff
(optional)
This value reflects how much redundancy (multicollinearity) among model
explanatory variables you will tolerate. When the VIF (Variance Inflation
Factor) value is higher than about 7.5, multicollinearity can make a model
unstable; consequently, 7.5 is the default value here. If you want your passing
models to have less redundancy, you would enter a smaller value, such as 5.0,
for this parameter.
Minimum Acceptable Jarque Bera p
value (optional)
The p-value returned by the Jarque-Bera diagnostic test indicates whether
the model residuals are normally distributed. If the p-value is statistically
significant (small), the model residuals are not normal and the model is
biased. Passing models should have large Jarque-Bera p-values. The default
minimum acceptable p-value is 0.1. Only models returning p-values larger than
this minimum will be considered passing. If you are having trouble finding unbiased
passing models, and decide to relax this criterion, you might enter a smaller
minimum p-value such as 0.05.
Minimum Acceptable Spatial
Autocorrelation p value (optional)
For models that pass all of the other search criteria, the Exploratory
Regression tool will check model residuals for spatial clustering using Global
Moran's I. When the p-value for this diagnostic test is statistically
significant (small), it indicates the model is very likely missing key
explanatory variables (it isn't telling the whole story). Unfortunately, if you
have spatial autocorrelation in your regression residuals, your model is
misspecified, so you cannot trust your results. Passing models should have
large p-values for this diagnostic test. The default minimum p-value is 0.1.
Only models returning p-values larger than this minimum will be considered
passing. If you are having trouble finding properly specified models because of
this diagnostic test, and decide to relax this search criteria, you might enter
a smaller minimum such as 0.05.
5.
Output Report File (optional) ملف
تقرير الإخراج (اختياري)
The report file contains
tool results, including details about any models found that passed all the
search criteria you entered. This output file also contains diagnostics to help
you fix common regression problems in the case that you don't find any passing
models.
يحتوي ملف التقرير على نتائج الأداة ، بما في ذلك
تفاصيل حول أي نماذج تم العثور عليها اجتازت جميع معايير البحث التي أدخلتها.
يحتوي ملف الإخراج هذا أيضًا على تشخيصات لمساعدتك في إصلاح مشكلات الانحدار
الشائعة في حالة عدم العثور على أي نماذج عابرة.
Output Results Table (optional)
The optional output table created containing the explanatory variables and
diagnostics for all of the models within the Coefficient p-value and VIF value
cutoffs.
Maximum Number of Explanatory
Variables (optional)
All models with explanatory variables up to the value entered here will be
assessed. If, for example, the Minimum Number of Explanatory Variables is 2 and
the Maximum Number of Explanatory Variables is 3, the Exploratory Regression
tool will try all models with every combination of two explanatory variables,
and all models with every combination of three explanatory variables.
Minimum Number of Explanatory
Variables (optional)
This value represents the minimum number of explanatory variables for
models evaluated. If, for example, the Minimum Number of Explanatory Variables
is 2 and the Maximum Number of Explanatory Variables is 3, the Exploratory
Regression tool will try all models with every combination of two explanatory
variables, and all models with every combination of three explanatory
variables.
Minimum Acceptable Adj R Squared
(optional)
This is the lowest Adjusted R-Squared value you consider a passing model.
If a model passes all of your other search criteria, but has an Adjusted
R-Squared value smaller than the value entered here, it will not show up as a
Passing Model in the Output Report File. Valid values for this parameter range
from 0.0 to 1.0. The default value is 0.05, indicating that passing models will
explain at least 50 percent of the variation in the dependent variable.
Maximum Coefficient p value Cutoff
(optional)
For each model evaluated, OLS computes explanatory variable coefficient
p-values. The cutoff p-value you enter here represents the confidence level you
require for all coefficients in the model in order to consider the model
passing. Small p-values reflect a stronger confidence level. Valid values for
this parameter range from 1.0 down to 0.0, but will most likely be 0.1, 0.05,
0.01, 0.001, and so on. The default value is 0.05, indicating passing models
will only contain explanatory variables whose coefficients are statistically at
the 95 percent confidence level (p-values smaller than 0.05). To relax this
default you would enter a larger p-value cutoff, such as 0.1. If you are
getting lots of passing models, you will likely want to make this search
criteria more stringent by decreasing the default p-value cutoff from 0.05 to
0.01 or smaller.
Maximum VIF Value Cutoff
(optional)
This value reflects how much redundancy (multicollinearity) among model
explanatory variables you will tolerate. When the VIF (Variance Inflation
Factor) value is higher than about 7.5, multicollinearity can make a model
unstable; consequently, 7.5 is the default value here. If you want your passing
models to have less redundancy, you would enter a smaller value, such as 5.0,
for this parameter.
Minimum Acceptable Jarque Bera p
value (optional)
The p-value returned by the Jarque-Bera diagnostic test indicates whether
the model residuals are normally distributed. If the p-value is statistically
significant (small), the model residuals are not normal and the model is
biased. Passing models should have large Jarque-Bera p-values. The default
minimum acceptable p-value is 0.1. Only models returning p-values larger than
this minimum will be considered passing. If you are having trouble finding
unbiased passing models, and decide to relax this criterion, you might enter a
smaller minimum p-value such as 0.05.
Minimum Acceptable Spatial
Autocorrelation p value (optional)
For models that pass all of the other search criteria, the Exploratory
Regression tool will check model residuals for spatial clustering using Global
Moran's I. When the p-value for this diagnostic test is statistically
significant (small), it indicates the model is very likely missing key
explanatory variables (it isn't telling the whole story). Unfortunately, if you
have spatial autocorrelation in your regression residuals, your model is
misspecified, so you cannot trust your results. Passing models should have
large p-values for this diagnostic test. The default minimum p-value is 0.1.
Only models returning p-values larger than this minimum will be considered passing.
If you are having trouble finding properly specified models because of this
diagnostic test, and decide to relax this search criteria, you might enter a
smaller minimum such as 0.05.
6.
Output Results Table (optional) جدول
نتائج المخرجات (اختياري)
The optional output
table created containing the explanatory variables and diagnostics for all of
the models within the Coefficient p-value and VIF value cutoffs.
تم إنشاء جدول الإخراج الاختياري الذي يحتوي على
المتغيرات التوضيحية والتشخيصات لجميع النماذج ضمن قيمة معامل p وقيمة
VIF.
Maximum Number of Explanatory
Variables (optional)
All models with explanatory variables up to the value entered here will be
assessed. If, for example, the Minimum Number of Explanatory Variables is 2 and
the Maximum Number of Explanatory Variables is 3, the Exploratory Regression
tool will try all models with every combination of two explanatory variables,
and all models with every combination of three explanatory variables.
Minimum Number of Explanatory
Variables (optional)
This value represents the minimum number of explanatory variables for
models evaluated. If, for example, the Minimum Number of Explanatory Variables
is 2 and the Maximum Number of Explanatory Variables is 3, the Exploratory
Regression tool will try all models with every combination of two explanatory
variables, and all models with every combination of three explanatory
variables.
Minimum Acceptable Adj R Squared
(optional)
This is the lowest Adjusted R-Squared value you consider a passing model.
If a model passes all of your other search criteria, but has an Adjusted
R-Squared value smaller than the value entered here, it will not show up as a
Passing Model in the Output Report File. Valid values for this parameter range
from 0.0 to 1.0. The default value is 0.05, indicating that passing models will
explain at least 50 percent of the variation in the dependent variable.
Maximum Coefficient p value Cutoff
(optional)
For each model evaluated, OLS computes explanatory variable coefficient
p-values. The cutoff p-value you enter here represents the confidence level you
require for all coefficients in the model in order to consider the model
passing. Small p-values reflect a stronger confidence level. Valid values for
this parameter range from 1.0 down to 0.0, but will most likely be 0.1, 0.05,
0.01, 0.001, and so on. The default value is 0.05, indicating passing models
will only contain explanatory variables whose coefficients are statistically at
the 95 percent confidence level (p-values smaller than 0.05). To relax this
default you would enter a larger p-value cutoff, such as 0.1. If you are
getting lots of passing models, you will likely want to make this search
criteria more stringent by decreasing the default p-value cutoff from 0.05 to
0.01 or smaller.
Maximum VIF Value Cutoff
(optional)
This value reflects how much redundancy (multicollinearity) among model
explanatory variables you will tolerate. When the VIF (Variance Inflation
Factor) value is higher than about 7.5, multicollinearity can make a model
unstable; consequently, 7.5 is the default value here. If you want your passing
models to have less redundancy, you would enter a smaller value, such as 5.0,
for this parameter.
Minimum Acceptable Jarque Bera p
value (optional)
The p-value returned by the Jarque-Bera diagnostic test indicates whether
the model residuals are normally distributed. If the p-value is statistically
significant (small), the model residuals are not normal and the model is
biased. Passing models should have large Jarque-Bera p-values. The default
minimum acceptable p-value is 0.1. Only models returning p-values larger than
this minimum will be considered passing. If you are having trouble finding
unbiased passing models, and decide to relax this criterion, you might enter a
smaller minimum p-value such as 0.05.
Minimum Acceptable Spatial
Autocorrelation p value (optional)
For models that pass all of the other search criteria, the Exploratory
Regression tool will check model residuals for spatial clustering using Global
Moran's I. When the p-value for this diagnostic test is statistically
significant (small), it indicates the model is very likely missing key
explanatory variables (it isn't telling the whole story). Unfortunately, if you
have spatial autocorrelation in your regression residuals, your model is
misspecified, so you cannot trust your results. Passing models should have
large p-values for this diagnostic test. The default minimum p-value is 0.1.
Only models returning p-values larger than this minimum will be considered
passing. If you are having trouble finding properly specified models because of
this diagnostic test, and decide to relax this search criteria, you might enter
a smaller minimum such as 0.05.
7.
Maximum Number of Explanatory Variables (optional) الحد الأقصى لعدد المتغيرات التوضيحية (اختياري)
All models with
explanatory variables up to the value entered here will be assessed. If, for
example, the Minimum Number of Explanatory Variables is 2 and the Maximum
Number of Explanatory Variables is 3, the Exploratory Regression tool will try
all models with every combination of two explanatory variables, and all models
with every combination of three explanatory variables.
سيتم تقييم جميع النماذج ذات المتغيرات التوضيحية
حتى القيمة التي تم إدخالها هنا. على سبيل المثال ، إذا كان الحد الأدنى لعدد
المتغيرات التوضيحية هو 2 وكان الحد الأقصى لعدد المتغيرات التوضيحية هو 3 ،
فستقوم أداة الانحدار الاستكشافي بتجربة جميع النماذج مع كل مجموعة من متغيرين
توضيحيين ، وجميع النماذج مع كل مجموعة من ثلاثة متغيرات توضيحية.
Minimum Number of Explanatory
Variables (optional)
This value represents the minimum number of explanatory variables for
models evaluated. If, for example, the Minimum Number of Explanatory Variables
is 2 and the Maximum Number of Explanatory Variables is 3, the Exploratory
Regression tool will try all models with every combination of two explanatory
variables, and all models with every combination of three explanatory
variables.
Minimum Acceptable Adj R Squared
(optional)
This is the lowest Adjusted R-Squared value you consider a passing model.
If a model passes all of your other search criteria, but has an Adjusted
R-Squared value smaller than the value entered here, it will not show up as a
Passing Model in the Output Report File. Valid values for this parameter range
from 0.0 to 1.0. The default value is 0.05, indicating that passing models will
explain at least 50 percent of the variation in the dependent variable.
Maximum Coefficient p value Cutoff
(optional)
For each model evaluated, OLS computes explanatory variable coefficient
p-values. The cutoff p-value you enter here represents the confidence level you
require for all coefficients in the model in order to consider the model
passing. Small p-values reflect a stronger confidence level. Valid values for
this parameter range from 1.0 down to 0.0, but will most likely be 0.1, 0.05,
0.01, 0.001, and so on. The default value is 0.05, indicating passing models
will only contain explanatory variables whose coefficients are statistically at
the 95 percent confidence level (p-values smaller than 0.05). To relax this
default you would enter a larger p-value cutoff, such as 0.1. If you are
getting lots of passing models, you will likely want to make this search
criteria more stringent by decreasing the default p-value cutoff from 0.05 to
0.01 or smaller.
Maximum VIF Value Cutoff
(optional)
This value reflects how much redundancy (multicollinearity) among model
explanatory variables you will tolerate. When the VIF (Variance Inflation Factor)
value is higher than about 7.5, multicollinearity can make a model unstable;
consequently, 7.5 is the default value here. If you want your passing models to
have less redundancy, you would enter a smaller value, such as 5.0, for this
parameter.
Minimum Acceptable Jarque Bera p
value (optional)
The p-value returned by the Jarque-Bera diagnostic test indicates whether
the model residuals are normally distributed. If the p-value is statistically
significant (small), the model residuals are not normal and the model is
biased. Passing models should have large Jarque-Bera p-values. The default
minimum acceptable p-value is 0.1. Only models returning p-values larger than
this minimum will be considered passing. If you are having trouble finding
unbiased passing models, and decide to relax this criterion, you might enter a
smaller minimum p-value such as 0.05.
Minimum Acceptable Spatial
Autocorrelation p value (optional)
For models that pass all of the other search criteria, the Exploratory
Regression tool will check model residuals for spatial clustering using Global
Moran's I. When the p-value for this diagnostic test is statistically
significant (small), it indicates the model is very likely missing key
explanatory variables (it isn't telling the whole story). Unfortunately, if you
have spatial autocorrelation in your regression residuals, your model is
misspecified, so you cannot trust your results. Passing models should have
large p-values for this diagnostic test. The default minimum p-value is 0.1. Only
models returning p-values larger than this minimum will be considered passing.
If you are having trouble finding properly specified models because of this
diagnostic test, and decide to relax this search criteria, you might enter a
smaller minimum such as 0.05.
8.
Minimum Number of Explanatory Variables (optional) الحد الأدنى لعدد المتغيرات التوضيحية (اختياري)
This value represents
the minimum number of explanatory variables for models evaluated. If, for
example, the Minimum Number of Explanatory Variables is 2 and the Maximum
Number of Explanatory Variables is 3, the Exploratory Regression tool will try
all models with every combination of two explanatory variables, and all models
with every combination of three explanatory variables.
تمثل هذه القيمة الحد الأدنى لعدد المتغيرات
التفسيرية للنماذج المقيمة. على سبيل المثال ، إذا كان الحد الأدنى لعدد المتغيرات
التوضيحية هو 2 وكان الحد الأقصى لعدد المتغيرات التوضيحية هو 3 ، فستقوم أداة
الانحدار الاستكشافي بتجربة جميع النماذج مع كل مجموعة من متغيرين توضيحيين ،
وجميع النماذج مع كل مجموعة من ثلاثة متغيرات توضيحية.
Minimum Acceptable Adj R Squared
(optional)
This is the lowest Adjusted R-Squared value you consider a passing model.
If a model passes all of your other search criteria, but has an Adjusted
R-Squared value smaller than the value entered here, it will not show up as a
Passing Model in the Output Report File. Valid values for this parameter range
from 0.0 to 1.0. The default value is 0.05, indicating that passing models will
explain at least 50 percent of the variation in the dependent variable.
Maximum Coefficient p value Cutoff
(optional)
For each model evaluated, OLS computes explanatory variable coefficient
p-values. The cutoff p-value you enter here represents the confidence level you
require for all coefficients in the model in order to consider the model
passing. Small p-values reflect a stronger confidence level. Valid values for
this parameter range from 1.0 down to 0.0, but will most likely be 0.1, 0.05,
0.01, 0.001, and so on. The default value is 0.05, indicating passing models
will only contain explanatory variables whose coefficients are statistically at
the 95 percent confidence level (p-values smaller than 0.05). To relax this
default you would enter a larger p-value cutoff, such as 0.1. If you are
getting lots of passing models, you will likely want to make this search
criteria more stringent by decreasing the default p-value cutoff from 0.05 to
0.01 or smaller.
Maximum VIF Value Cutoff
(optional)
This value reflects how much redundancy (multicollinearity) among model
explanatory variables you will tolerate. When the VIF (Variance Inflation
Factor) value is higher than about 7.5, multicollinearity can make a model
unstable; consequently, 7.5 is the default value here. If you want your passing
models to have less redundancy, you would enter a smaller value, such as 5.0,
for this parameter.
Minimum Acceptable Jarque Bera p
value (optional)
The p-value returned by the Jarque-Bera diagnostic test indicates whether
the model residuals are normally distributed. If the p-value is statistically
significant (small), the model residuals are not normal and the model is
biased. Passing models should have large Jarque-Bera p-values. The default
minimum acceptable p-value is 0.1. Only models returning p-values larger than
this minimum will be considered passing. If you are having trouble finding
unbiased passing models, and decide to relax this criterion, you might enter a
smaller minimum p-value such as 0.05.
Minimum Acceptable Spatial Autocorrelation
p value (optional)
For models that pass all of the other search criteria, the Exploratory
Regression tool will check model residuals for spatial clustering using Global
Moran's I. When the p-value for this diagnostic test is statistically significant
(small), it indicates the model is very likely missing key explanatory
variables (it isn't telling the whole story). Unfortunately, if you have
spatial autocorrelation in your regression residuals, your model is
misspecified, so you cannot trust your results. Passing models should have
large p-values for this diagnostic test. The default minimum p-value is 0.1.
Only models returning p-values larger than this minimum will be considered
passing. If you are having trouble finding properly specified models because of
this diagnostic test, and decide to relax this search criteria, you might enter
a smaller minimum such as 0.05.
9.
Minimum Acceptable Adj R Squared (optional) الحد الأدنى المقبول من Adj R تربيع (اختياري)
This is the lowest
Adjusted R-Squared value you consider a passing model. If a model passes all of
your other search criteria, but has an Adjusted R-Squared value smaller than
the value entered here, it will not show up as a Passing Model in the Output Report
File. Valid values for this parameter range from 0.0 to 1.0. The default value
is 0.05, indicating that passing models will explain at least 50 percent of the
variation in the dependent variable.
هذه هي أدنى قيمة R-Squared معدلة تعتبرها نموذجًا ناجحًا. إذا اجتاز النموذج جميع معايير
البحث الأخرى الخاصة بك ، ولكن لديه قيمة R-Squared معدلة
أصغر من القيمة التي تم إدخالها هنا ، فلن يظهر كنموذج تمرير في ملف تقرير
الإخراج. تتراوح القيم الصالحة لهذه المعلمة من 0.0 إلى 1.0. القيمة الافتراضية هي
0.05 ، مما يشير إلى أن النماذج العابرة سوف تشرح ما لا يقل عن 50 في المائة من
التباين في المتغير التابع.
Maximum Coefficient p value Cutoff
(optional)
For each model evaluated, OLS computes explanatory variable coefficient
p-values. The cutoff p-value you enter here represents the confidence level you
require for all coefficients in the model in order to consider the model
passing. Small p-values reflect a stronger confidence level. Valid values for
this parameter range from 1.0 down to 0.0, but will most likely be 0.1, 0.05,
0.01, 0.001, and so on. The default value is 0.05, indicating passing models
will only contain explanatory variables whose coefficients are statistically at
the 95 percent confidence level (p-values smaller than 0.05). To relax this
default you would enter a larger p-value cutoff, such as 0.1. If you are
getting lots of passing models, you will likely want to make this search
criteria more stringent by decreasing the default p-value cutoff from 0.05 to
0.01 or smaller.
Maximum VIF Value Cutoff (optional)
This value reflects how much redundancy (multicollinearity) among model
explanatory variables you will tolerate. When the VIF (Variance Inflation
Factor) value is higher than about 7.5, multicollinearity can make a model
unstable; consequently, 7.5 is the default value here. If you want your passing
models to have less redundancy, you would enter a smaller value, such as 5.0,
for this parameter.
Minimum Acceptable Jarque Bera p
value (optional)
The p-value returned by the Jarque-Bera diagnostic test indicates whether
the model residuals are normally distributed. If the p-value is statistically
significant (small), the model residuals are not normal and the model is
biased. Passing models should have large Jarque-Bera p-values. The default
minimum acceptable p-value is 0.1. Only models returning p-values larger than
this minimum will be considered passing. If you are having trouble finding
unbiased passing models, and decide to relax this criterion, you might enter a
smaller minimum p-value such as 0.05.
Minimum Acceptable Spatial
Autocorrelation p value (optional)
For models that pass all of the other search criteria, the Exploratory
Regression tool will check model residuals for spatial clustering using Global
Moran's I. When the p-value for this diagnostic test is statistically
significant (small), it indicates the model is very likely missing key
explanatory variables (it isn't telling the whole story). Unfortunately, if you
have spatial autocorrelation in your regression residuals, your model is misspecified,
so you cannot trust your results. Passing models should have large p-values for
this diagnostic test. The default minimum p-value is 0.1. Only models returning
p-values larger than this minimum will be considered passing. If you are having
trouble finding properly specified models because of this diagnostic test, and
decide to relax this search criteria, you might enter a smaller minimum such as
0.05.
10.
Maximum Coefficient p value Cutoff (optional) الحد الأقصى لقيمة p للمعامل
(اختياري)
For each model
evaluated, OLS computes explanatory variable coefficient p-values. The cutoff
p-value you enter here represents the confidence level you require for all
coefficients in the model in order to consider the model passing. Small
p-values reflect a stronger confidence level. Valid values for this parameter
range from 1.0 down to 0.0, but will most likely be 0.1, 0.05, 0.01, 0.001, and
so on. The default value is 0.05, indicating passing models will only contain
explanatory variables whose coefficients are statistically at the 95 percent
confidence level (p-values smaller than 0.05). To relax this default you would
enter a larger p-value cutoff, such as 0.1. If you are getting lots of passing
models, you will likely want to make this search criteria more stringent by
decreasing the default p-value cutoff from 0.05 to 0.01 or smaller.
لكل نموذج يتم تقييمه ، يحسب OLS قيم p
لمعامل المتغير التوضيحي. تمثل قيمة p-cutoff التي
تدخلها هنا مستوى الثقة الذي تطلبه لجميع المعاملات في النموذج من أجل النظر في
اجتياز النموذج. تعكس قيم p
الصغيرة مستوى ثقة أقوى. تتراوح القيم الصالحة لهذه المعلمة من 1.0 إلى 0.0 ، ولكن
من المرجح أن تكون 0.1 و 0.05 و 0.01 و 0.001 وهكذا. القيمة الافتراضية هي 0.05 ،
مما يشير إلى أن النماذج العابرة ستحتوي فقط على متغيرات توضيحية تكون معاملاتها
إحصائيًا عند مستوى ثقة 95 بالمائة (قيم p أصغر
من 0.05). لتخفيف هذا الوضع الافتراضي ، ستدخل حدًا أكبر للقيمة الاحتمالية ، مثل
0.1. إذا كنت تحصل على الكثير من نماذج النجاح ، فمن المحتمل أن ترغب في جعل
معايير البحث هذه أكثر صرامة عن طريق تقليل الحد الافتراضي للقيمة الاحتمالية من
0.05 إلى 0.01 أو أصغر.
Maximum VIF Value Cutoff
(optional)
This value reflects how much redundancy (multicollinearity) among model
explanatory variables you will tolerate. When the VIF (Variance Inflation
Factor) value is higher than about 7.5, multicollinearity can make a model
unstable; consequently, 7.5 is the default value here. If you want your passing
models to have less redundancy, you would enter a smaller value, such as 5.0,
for this parameter.
Minimum Acceptable Jarque Bera p
value (optional)
The p-value returned by the Jarque-Bera diagnostic test indicates whether
the model residuals are normally distributed. If the p-value is statistically
significant (small), the model residuals are not normal and the model is biased.
Passing models should have large Jarque-Bera p-values. The default minimum
acceptable p-value is 0.1. Only models returning p-values larger than this
minimum will be considered passing. If you are having trouble finding unbiased
passing models, and decide to relax this criterion, you might enter a smaller
minimum p-value such as 0.05.
Minimum Acceptable Spatial
Autocorrelation p value (optional)
For models that pass all of the other search criteria, the Exploratory
Regression tool will check model residuals for spatial clustering using Global
Moran's I. When the p-value for this diagnostic test is statistically
significant (small), it indicates the model is very likely missing key
explanatory variables (it isn't telling the whole story). Unfortunately, if you
have spatial autocorrelation in your regression residuals, your model is
misspecified, so you cannot trust your results. Passing models should have
large p-values for this diagnostic test. The default minimum p-value is 0.1.
Only models returning p-values larger than this minimum will be considered
passing. If you are having trouble finding properly specified models because of
this diagnostic test, and decide to relax this search criteria, you might enter
a smaller minimum such as 0.05.
11.
Maximum VIF Value Cutoff (optional) الحد الأقصى لقطع قيمة VIF (اختياري)
This value reflects how
much redundancy (multicollinearity) among model explanatory variables you will
tolerate. When the VIF (Variance Inflation Factor) value is higher than about
7.5, multicollinearity can make a model unstable; consequently, 7.5 is the
default value here. If you want your passing models to have less redundancy,
you would enter a smaller value, such as 5.0, for this parameter.
تعكس هذه القيمة مقدار التكرار (العلاقة الخطية
المتعددة) بين المتغيرات التوضيحية للنموذج التي ستتحملها. عندما تكون قيمة VIF (عامل
تضخم التباين) أعلى من 7.5 تقريبًا ، يمكن أن تجعل العلاقة الخطية المتعددة
النموذج غير مستقر ؛ وبالتالي ، 7.5 هي القيمة الافتراضية هنا. إذا كنت تريد تقليل
التكرار في النماذج التي تم اجتيازها ، فيمكنك إدخال قيمة أصغر ، مثل 5.0 ، لهذه
المعلمة.
Minimum Acceptable Jarque Bera p
value (optional)
The p-value returned by the Jarque-Bera diagnostic test indicates whether
the model residuals are normally distributed. If the p-value is statistically
significant (small), the model residuals are not normal and the model is
biased. Passing models should have large Jarque-Bera p-values. The default
minimum acceptable p-value is 0.1. Only models returning p-values larger than
this minimum will be considered passing. If you are having trouble finding
unbiased passing models, and decide to relax this criterion, you might enter a
smaller minimum p-value such as 0.05.
Minimum Acceptable Spatial
Autocorrelation p value (optional)
For models that pass all of the other search criteria, the Exploratory
Regression tool will check model residuals for spatial clustering using Global
Moran's I. When the p-value for this diagnostic test is statistically
significant (small), it indicates the model is very likely missing key
explanatory variables (it isn't telling the whole story). Unfortunately, if you
have spatial autocorrelation in your regression residuals, your model is
misspecified, so you cannot trust your results. Passing models should have
large p-values for this diagnostic test. The default minimum p-value is 0.1.
Only models returning p-values larger than this minimum will be considered
passing. If you are having trouble finding properly specified models because of
this diagnostic test, and decide to relax this search criteria, you might enter
a smaller minimum such as 0.05.
12.
Minimum Acceptable Jarque Bera p value (optional) الحد الأدنى المقبول لقيمة Jarque Bera p (اختياري)
The p-value returned by
the Jarque-Bera diagnostic test indicates whether the model residuals are
normally distributed. If the p-value is statistically significant (small), the
model residuals are not normal and the model is biased. Passing models should
have large Jarque-Bera p-values. The default minimum acceptable p-value is 0.1.
Only models returning p-values larger than this minimum will be considered
passing. If you are having trouble finding unbiased passing models, and decide
to relax this criterion, you might enter a smaller minimum p-value such as
0.05.
تشير القيمة p التي
تم إرجاعها بواسطة اختبار تشخيص Jarque-Bera إلى
ما إذا كانت بقايا النموذج موزعة بشكل طبيعي. إذا كانت القيمة p ذات
دلالة إحصائية (صغيرة) ، فإن بقايا النموذج ليست طبيعية ويكون النموذج متحيزًا.
يجب أن تحتوي نماذج التمرير على قيم p Jarque-Bera
كبيرة. الحد الأدنى الافتراضي للقيمة الاحتمالية المقبولة هو 0.1. فقط النماذج
التي تُرجع قيم p أكبر من هذا الحد الأدنى سيتم اعتبارها عابرة. إذا كنت تواجه
مشكلة في العثور على نماذج اجتياز غير متحيزة ، وقررت تخفيف هذا المعيار ، فيمكنك
إدخال قيمة p دنيا أصغر مثل 0.05.
Minimum Acceptable Spatial
Autocorrelation p value (optional)
For models that pass all of the other search criteria, the Exploratory
Regression tool will check model residuals for spatial clustering using Global
Moran's I. When the p-value for this diagnostic test is statistically
significant (small), it indicates the model is very likely missing key
explanatory variables (it isn't telling the whole story). Unfortunately, if you
have spatial autocorrelation in your regression residuals, your model is
misspecified, so you cannot trust your results. Passing models should have
large p-values for this diagnostic test. The default minimum p-value is 0.1.
Only models returning p-values larger than this minimum will be considered passing.
If you are having trouble finding properly specified models because of this
diagnostic test, and decide to relax this search criteria, you might enter a
smaller minimum such as 0.05.
13.
Minimum Acceptable Spatial Autocorrelation p value
(optional) الحد الأدنى المقبول للقيمة
p للارتباط التلقائي المكاني (اختياري)
For models that pass all
of the other search criteria, the Exploratory Regression tool will check model
residuals for spatial clustering using Global Moran's I. When the p-value for
this diagnostic test is statistically significant (small), it indicates the
model is very likely missing key explanatory variables (it isn't telling the
whole story). Unfortunately, if you have spatial autocorrelation in your
regression residuals, your model is misspecified, so you cannot trust your
results. Passing models should have large p-values for this diagnostic test.
The default minimum p-value is 0.1. Only models returning p-values larger than
this minimum will be considered passing. If you are having trouble finding
properly specified models because of this diagnostic test, and decide to relax
this search criteria, you might enter a smaller minimum such as 0.05.
بالنسبة إلى النماذج التي تجتاز جميع معايير البحث
الأخرى ، ستتحقق أداة الانحدار الاستكشافي من مخلفات النموذج للتجميع المكاني
باستخدام Global Moran I. عندما تكون القيمة p لهذا
الاختبار التشخيصي مهمة من الناحية الإحصائية (صغيرة) ، فهذا يشير إلى أن النموذج
محتمل جدًا عدم وجود المتغيرات التفسيرية الرئيسية (لا تروي القصة كاملة). لسوء
الحظ ، إذا كان لديك ارتباط تلقائي مكاني في بقايا الانحدار ، فإن نموذجك محدد
بشكل خاطئ ، لذلك لا يمكنك الوثوق بنتائجك. يجب أن تحتوي نماذج النجاح على قيم p كبيرة
لهذا الاختبار التشخيصي. الحد الأدنى الافتراضي لقيمة p هو
0.1. فقط النماذج التي تُرجع قيم p أكبر
من هذا الحد الأدنى سيتم اعتبارها عابرة. إذا كنت تواجه مشكلة في العثور على نماذج
محددة بشكل صحيح بسبب هذا الاختبار التشخيصي ، وقررت تخفيف معايير البحث هذه ،
يمكنك إدخال حد أدنى أصغر مثل 0.05.
اليك صفحه ومجموعة على الفيس بوك لتعلم أكثر بما يخص نظم المعلومات الجغرافية (GIS) و برنامج ArcGIS Pro من خلال هذه الروابط:
مجموعة على الفيس بوك
ArcGIS Pro من
هنا.
مجموعة على الفيس بوك
GIS for WE - ArcGIS Pro من
هنا.صفحة الفيس بوك
GIS for WE من
هنا.
تعليقات
إرسال تعليق